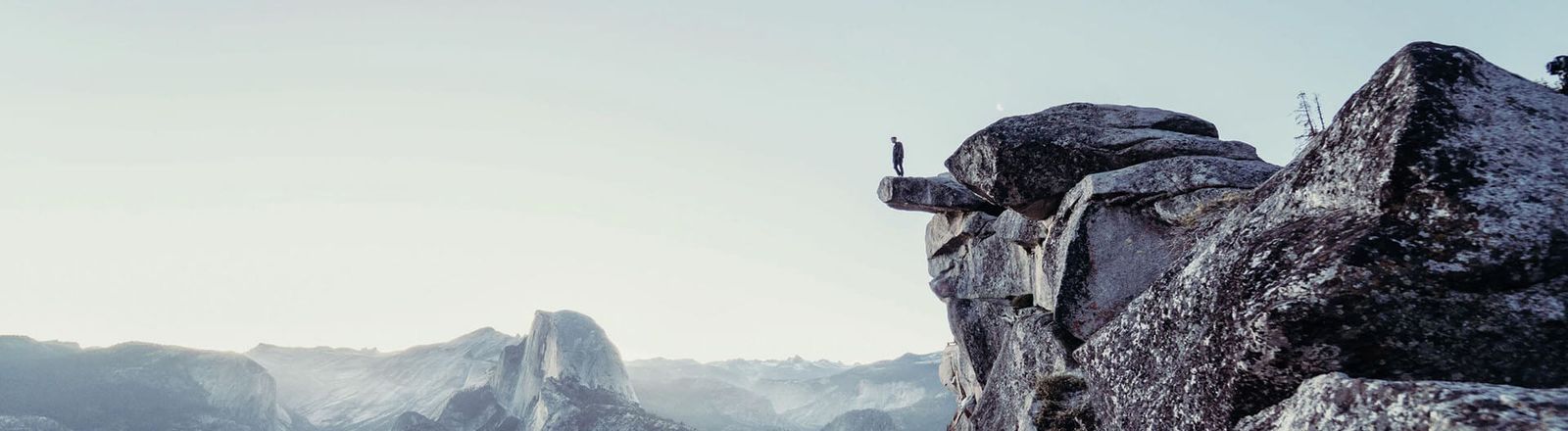
04/14/2021 · Adam Tachner
Brave Thinking
I started out in the 1990s as a patent attorney, and over the years I’ve worked with some very, very bright inventors. I love doing IP work with cutting-edge technologists — you walk in the room, check your ego at the door, and learn from experts in their fields sharing visionary ideas.
After six years of law firm IP practice, I wanted to get closer to the handle of the business whip. That’s when I got a call from the outstanding VC, Andy Rappaport. Andy’s firm August Capital had funded a new venture that would later come to be known as Atheros Communications. Andy pitched me to join the leadership team of a company that would help make WiFi a globally-known, household word. Even though I was just weeks from becoming a law firm partner, I took the plunge — Brave thinking? Reckless abandon? — and never looked back. I was employee number 34 or 35; to be sure, I’d have to ask the other guy who started that day. By the time we sold Atheros to Qualcomm, we had grown the team to nearly 2,000 people with annual revenue of $1 billion.
I’d have loved to jump into another silicon startup right away, but it was 2011, the middle of a quiet time for semiconductor startup funding. Returns even on successful companies were muted, so there was less money being invested in semis. It seemed there was less appetite for investments in core technology generally – especially the exciting, physics-driven, tangible stuff. So, instead of another startup, I joined InvenSense, an amazing fabless MEMS company that we built up and sold to TDK. At InvenSense I ran Corporate Development as well as legal; we searched out unique AI/ML assets in sensor data management and fusion.
Then a former boss recruited me to join Google, to help lead and spin-out an Alphabet company. While that deal never happened, I did observe life flowing back into silicon startups. I looked into getting back in, and met with several AI/ML founders and CEOs.
I think it’s fair to generalize that there are two ways of architecting compute solutions, especially for AI. The first is to increment on existing architectures. That’s what everyone seemed to be doing. Then I checked in with a friend – one of Google’s top engineers and AI thought leaders – who had sponsored the TPU, Google’s then-new and now-dominant internal compute platform. When I asked my friend who else I should talk to in AI/ML silicon, he didn’t miss a beat: Jonathan Ross had been the divergent thinker behind the TPU. “Jonathan left Google to start Groq. Let me introduce you.”
Groq started from a clean slate and was indeed doing something different–have I mentioned that brave thinking is my favorite kind? In 2019, I joined. I am employee number 50 or 51; to be sure, I’d have to ask the other guy who started that day.
A clean slate approach allows Groq to actually solve customer problems at the root, not just apply available, sub-optimal architectures that are tweaked, adapted and compromised. You could design a car by starting with the engine, the brakes, and the mechanics of it, or you could start with how you want driving the car to feel. Most people design a chip by starting from the hardware, but at Groq we started with a clean slate and focused on the software compiler. That is, we started from the user’s perspective. Batch1 thinking resulted from a clean slate; instead of asking customers to choose between latency and throughput, Groq enabled both. That approach also led to determinism at scale via a high-speed chip-to-chip network, which eases programming and bring-up of new models, even at scale, and without so much expensive switching equipment.
Fast forward to 2021: Groq has put together a top-quality group of investors to close its Series C fundraise, bringing total funding to $367 million, and $300 million raised since the second-half of 2020 — a direct result of strong customer endorsement. We’ve doubled our team size since July, and will do so again within a year.
Despite considerable customer and investor traction, some people think Groq’s solution is too good to be true. Perhaps they are stuck in the model of throwing thousands of custom coders at every new challenge that comes along. Maybe they think massive engineering teams are a moat that everyone else must cross.
I wonder whether that moat is really a cage with golden bars, with the incumbents and their customers stuck inside, watching the evolution of AI and ML flow by. As AI evolves faster and faster, what amount of people can you throw at these new problems to stay ahead without a compilable architecture? Is that effort any less futile than Yahoo trying to manually index the internet to compete with Google’s PageRank? Recently, AI models started writing other AI models. How does custom hand coding stay ahead of that?
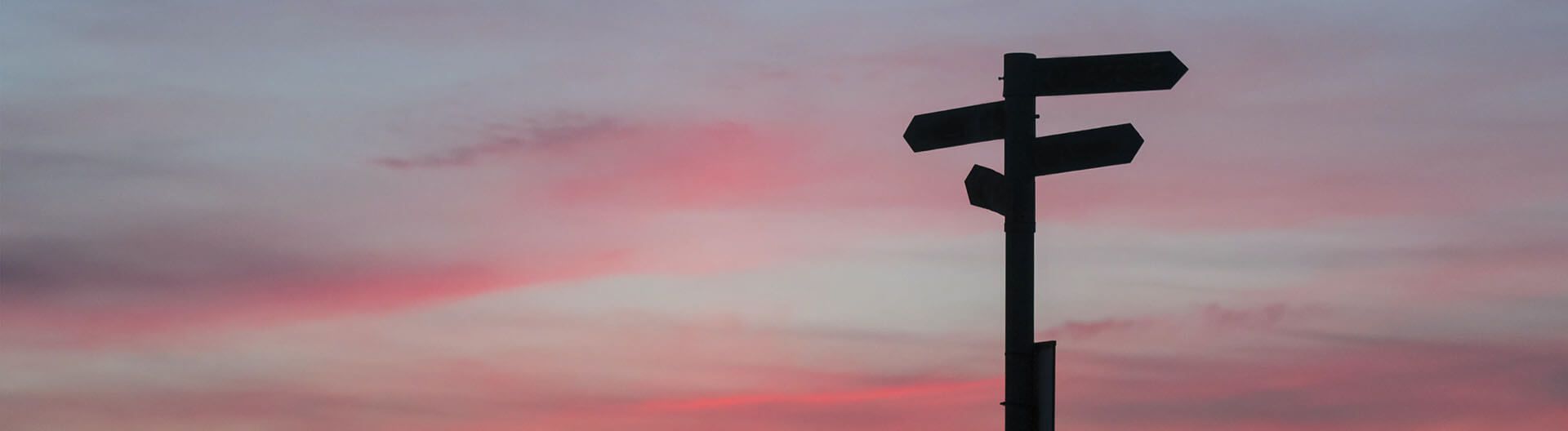
You could say Groq has a brave, almost reckless way of thinking. Or maybe the industry has hit a turning point, and only those willing to break away from the old ways can forge the new path. We’re avoiding the old rules with a first principles, foundational approach. And that‘s a big part of what makes it so amazing to work at Groq.
That’s what I told my longtime-friend Andy Rappaport – the one who helped recruit me into Atheros. When I first called Andy about Groq early last year, he was very happily retired from semis, even though many people had been trying for years to pull him into their projects. He had politely refused one offer after another. But when Andy saw what we were doing at Groq, he couldn’t resist the vision, and joined our Board of Directors. Brave thinking, indeed, has brought us full circle.